The 5 Pillars to Successful Data Quality Management
The 5 Pillars of Data Quality Management
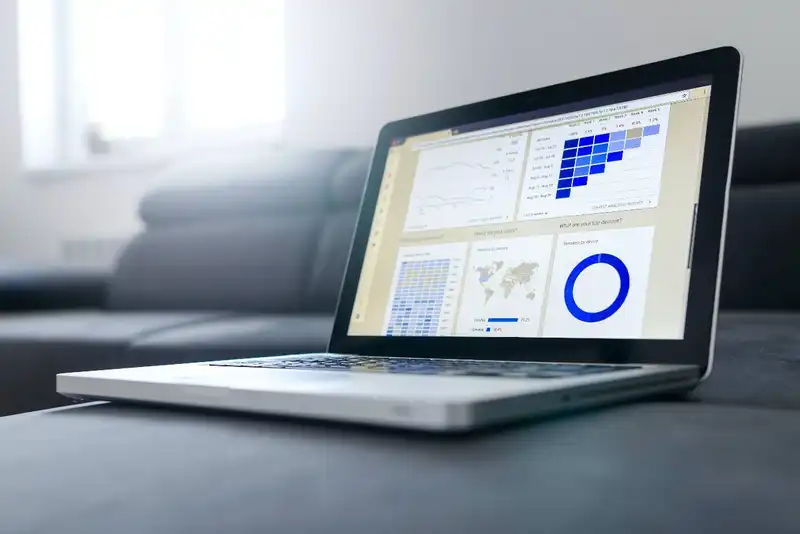
What exactly is data quality management? Quality management is a set of quality standards that aim at housing quality data. In other words, a quality management program uses different procedures and quality tools to ensure all collected information is reliable, accurate, and meets quality standards.
An organization acquires real-time new data that is used to create good business intelligence. Quality control managers need to oversee all of the big data to pinpoint any quality issue and eliminate data errors. Employees and executives use data to improve decision-making and problem-solving. But if the information isn't accurate, decisions won't be either.
Quality management data specialists employ a specific quality improvement strategy to ensure each data set is reliable. Management DQM processes ensure a business is able to handle any quality problems with ease whenever they arise. When employees and executives can extract valuable insights from good master data, they can pinpoint inefficiencies and eliminate bottlenecks.
From customer relationships to managing the supply chain, effective data management and data analytics can improve a company's operational effectiveness. With data stewards and data cleansing, IT can generate a self-service data warehouse that holds all company information. Easy to access and simple to use, business users, harness information to optimize business processes every day.
1. Management Program The People
Data quality tools and other software systems are only effective when people know how to operate them. Machine learning and other techniques are great to drill down into organization data, but only if humans are supervising the process. Businesses need to fill various roles to ensure the data quality management process works properly. These include
- Program Manager - A qualified leader that is responsible enough to oversee any good data projects or company initiatives. This individual supervises any data lineage issues, scope, budget, and requirements to carry out each task. At the forefront of his/her mind should be quality issues and receiving a return on investment.
- Change Manager- This individual organizes data management initiatives. He/she also identifies any necessary information about data platforms or other business intelligence solutions.
- Data Analyst - Data analysts identify what the organization needs from its defining data and how it plans to use different data sets. Any set data needs are then turned into data models and quality tools. The analyst communicates any of these rules to development team members.
2. Improve Data Data Profiling
The data lifecycle can't survive without data profiling. Profiling develops an understanding of current data assets and quality standards and whether they are meeting company objectives. This may include data integrity rules, data accuracy requirements, or other data entry and warehousing issues. It is a starting point to implement quality rules, improve data, and eliminate bad data. Data profilers look for accuracy in data, any discrepancies, or duplicate information.
3. Define and Measure Data Quality
Quality business rules are based on an organization's specific needs. Data quality rules include all of the technical standards that data stewards must comply with. This eliminates the root cause of any bad data and prevents it from harming the entire system.
Established rules naturally resolve most quality control issues. When employees or owners use both data quality rules and a BI platform, they can easily forecast patterns and generate reports.
4. Management Data Reporting
Reporting eliminates and records any duplicate or corrupt data. Once these issues are recorded, analysts can codify them to pinpoint trends. Any aggregated bad data is typically analyzed to see which rules or sources caused the problem.
Users can use data reporting or a dashboard to explain all of the quality of information and any concern areas. When businesses regularly check data quality with reporting tools, they eliminate any recurring data quality issues. This improves decision-making across the organization and increases the return on investment.
5. Ensuring Data Repair
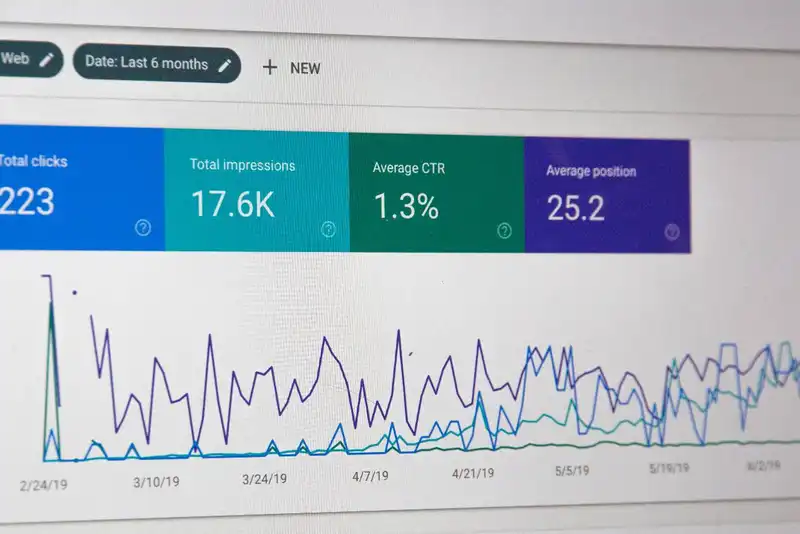
Data repair determines the most optimal way to fix or reorganize data and how to do it efficiently. It's critical to pinpoint why the issues happened, what platform/system it occurred in, and where it started. When these questions are answered, the organization can determine a remediation course of action.
Any processes that were based on corrupt information need to be restarted. The business should also review any data quality standards and rules to determine if anything needs to be changed or fixed. When all collected information is high quality and secure, every other workflow and process will improve too.
Key Takeaways for Data Management
In conclusion, here are the data quality assessment pillars
- People in a data quality management DQM include everyone who uses data internally or externally. The individuals involved in this process include a program manager, business rule setter, and data analyst.
- Data profiling increases the understanding of all collected information and determines whether it is meeting company standards.
- Data quality managers eliminate any inaccurate or corrupt information to improve the quality of all organization data. Data reporting aggregates any information about data quality and quality dimensions so users can present it to the remediation team.
- Data repair specialists fix any poor rules or networks that cause inaccurate data results. This will improve decision-making and make it easier for employees to perform their job.