5 Stages in Data Lifecycle Management
Insight into Data Lifecycle Management
Owners that research IT systems and policies have probably read about lifecycle management DLM. Similar to the majority of IT-related activities, businesses don't simply create and deliver data to clients. Unfortunately, the process is much more intricate and complicated.
The data a business collects impacts each business unit, worker, owner, client, and business user. Because of how importance data is to the business environment, data procedures must align with each phase of the lifecycle. Read ahead to find out what cycle management is and what occurs throughout each stage of the lifecycle.
The 5 Main Stages in Data Lifecycle Management Process
Just as each living organism goes through a series of changes throughout its lifetime, so does data. Gathered company data didn't start as valuable or interesting. It took a series of tools, cleaning processes, and techniques to turn management data into something usable and helpful.
Digital transformation and easy data access are changing the way a small business approaches data collection, storage data, and data loss. Each piece of new data is potentially critical in an era where competitors leverage business data to their advantage.
To ensure lifecycle data is high-quality and useful for business intelligence, it's important to understand data lifecycle management. Here is how collected information evolves throughout the data life cycle
1. Creating Data
The first data phase of lifecycle management data is the data creation stage. The data a business creates can be in different formats such as a customer relationship management system, cloud data, or social media platforms. There are three ways that an organization creates data. These include
- Acquisition- Gather external existing information
- Entry- Employees input new information internally
- Capture- Devices capture data from different sectors of a business
2. Data Storage
When a business generates data, it then stores it and enacts data security measures to prevent data loss. There must be a vigorous backup and loss prevention strategy in place to make sure the data is secure throughout its life cycle. It's critical for IT personnel to aim to protect both structured and unstructured data in the data cloud and remember compliance requirements.
It's essential to establish a data retention policy that includes data archiving and other lifecycle management processes. IT should not store redundant information as users may generate faulty insights and make poor business decisions. Data security measures are critical throughout the entirety of the data life phases in data management.
3. Data Use
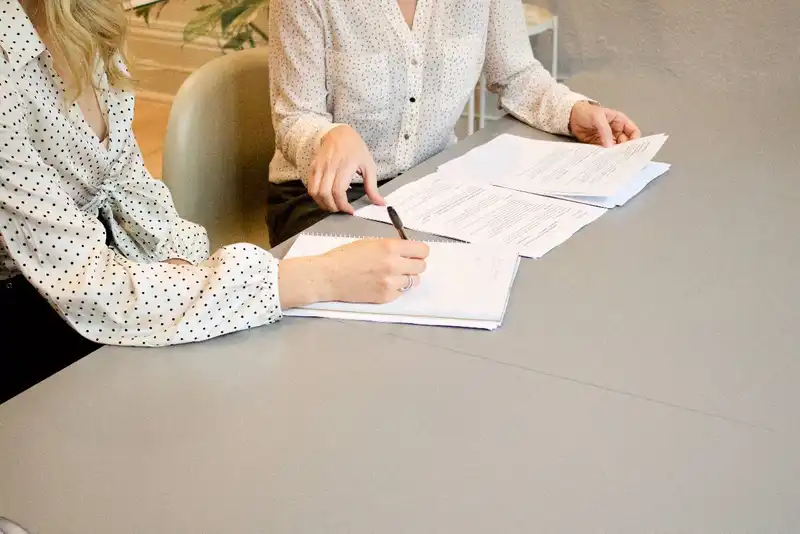
In the usage phase of the management data lifecycle, information is the foundation for decision-making across the business. Users can retrieve phase data from management software, process it, change it, and save it to their applications.
Best practices include using an audit trail to ensure that all changes to data are referenceable. In a data governance policy, specialists can determine which information should be available to customers and customer support. Sometimes specialists mistakenly limit the data available to users too much. Users may require greater access to information for analysis and reporting use cases. Developers should work with executives to ensure data usage policies align with company needs.
4. Data Archive
A data archive refers to the copy of the information to a storable location. Developers do this because too often, users don't think they need certain data. Until they do. Archiving even the most mundane information is essential because certain data may be useful way down the road.
Archiving also requires developers to remove unnecessary or old information from the production databases. It is then stored in a separate location so users can't easily access it. There is no maintenance on any of the archived data unless it is placed back in the active database.
5. Data Destruction
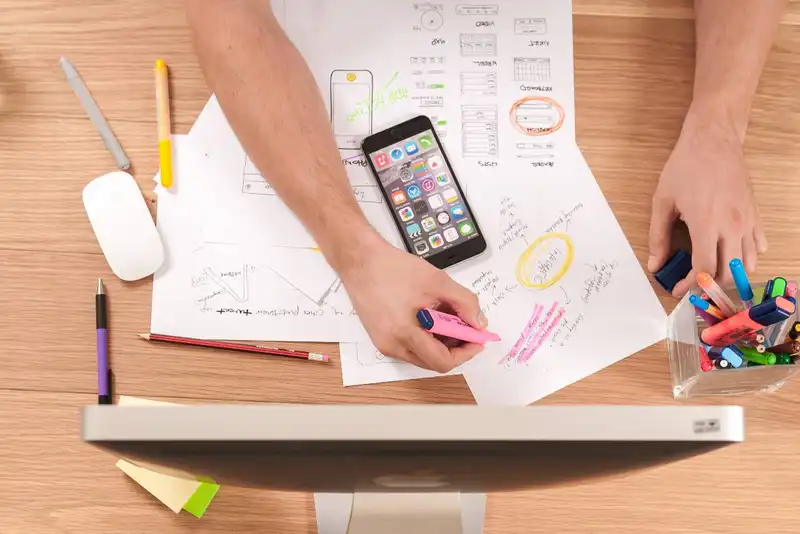
Because organizations collect new information each day, it's not possible to save data longer than necessary. While cloud storage is typically less expensive, it's still costly to house all the information users collect.
Furthermore, there are compliance concerns that business leaders need to consider. Inaccurate or outdated data can cause poor decisions or mistakes that result in non-compliance. Developers purge information from an archive database.
Governance policies can determine when and how often to destroy information. It can be difficult to know if the information is really destroyed or if it just appears to be. It's also critical to ensure that any destroyed data is authorized for deletion in alignment with compliance standards.
Key Takeaways About Data Lifecycle Management
In conclusion, here is what to remember about data lifecycle management data
- To create data, developers should get data outside the organization, input DLM data manually, and retrieve it from different devices within the business. To store data, developers should implement data protection policies, retain it, and eliminate any duplicate information.
- Developers should create an audit trail for all information to ensure it doesn't get lost. They can also establish policies for users to manage data, find data stored, and save it to their applications.
- Developers must archive information even if users don't think they need it. They must remove unnecessary value data from productive databases and store it in a separate location to ensure data loss prevention.
- Businesses must destroy data after some time because it is expensive to store. Inaccurate or outdated information can also interfere with regulatory compliance. All data destruction policies should align with company standards.