Data Analysis Methods, Types, and Best Practices
In recent years, digital and technological advancements have provided so many efficiency and productivity tools for business and industry that executives and managers are sometimes hard-pressed to keep up. Data-driven businesses have given rise to new data analysis paradigms and hardware improvements in technology, adding to the tsunami of solutions being brought to market every year.
Organizations are generating dizzying volumes of data on a daily basis, with companies in some industries streaming tens of thousands of data points per second. This was simply not technically feasible once upon a time, but now, it has afforded organizations the opportunity to dramatically improve all of their operations.
While big companies like Microsoft and Google implement big data in their decision-making (and lots of it, due to their size), small businesses, retailers, medical organizations, government agencies and a host of other industries are also employing data analysis.
Like the process of becoming a data-driven organization, the methodologies behind data analysis is are difficult to adapt to. It's simply a matter of becoming acquainted with the key principles and concepts, followed by putting a few simple ideas into practice.
Data Analysis- A Definition
So, what exactly is data analysis? In short, data analysis is a process of evaluating data using one or more analytical tools. In so doing, the relevance of the data provided to the objectives and goals of the organization can be determined. Data from different sources is gathered, examined, and finally analyzed to reach a conclusion or conclusions.
There are many specific data analysis methods; some of these include areas like data mining, text analytics, business intelligence, and data visualizations.
Prior to delving into data analysis, however, it is essential to understand the two types of data, and the differences between them.
Types of Data
The first step in understanding data analysis is in recognizing these two classifications. Qualitative data and quantitative data are distinctly different, but they encompass all data that will be analyzed in an organization.
Qualitative data is comprised of non-statistical information and tends to be loosely structured. It is categorized based on various identifiers, rather than being used to generate hard numbers for output. Qualitative data usually answers basic questions and can give rise to more specific questions.
Investigative in nature, qualitative data is used in developing theories, hypotheses, and initial insights.
Qualitative data is usually aggregated through documents, images, transcripts, audio and video media, observations, notes, and information from focus groups.
Quantitative data is hard data. More rigidly-defined, it is statistical and is typically represented using numbers and other values, making it a more ready candidate for data analysis.
Quantitative data can be broken down further into two subsets- Discrete data and continuous data.
- Discrete data is distinctive, singular information that cannot be broken down into lesser parts. Examples of discrete data include the amount of money in a tip jar, or how many smart phones were sold in a given year.
- Continuous data is data that can be broken down into smaller parts, or data that changes on an ongoing basis. Examples of continuous data might include the speed of an airliner over the course of a flight, or the time it takes to complete a repetitive task.
Data Analysis Methods
Having determined what data analysis is and the classes of data, the methods by which data will be analyzed need to be identified. Familiarization with these will be crucial in arriving at qualitative insights, the direction of quantitative research and will impart a clear roadmap for the organization's data analysis.
While the timing of implementation will become self-evident based on the needs of the organization, it will be helpful to bear in mind that these are methods to incorporate into data analysis practices, rather than steps that should be taken in precise order.
Determine Direction
This process involves engaging stakeholders in the organization, determining strategic goals, and arriving at an understanding among stakeholders and decision-makers as to the kinds of insights (derived from data) that will best serve the organization.
Establish Parameters
One of the most important parts of the process, it is nevertheless a straightforward one.
Establishing parameters essentially involves determining the questions that will need to be answered in order to glean the best insights from the data.
It's been said that data will only be as good as the questions asked. Some experts suggest that the best approach is to identify the pain points in an organization first, as these are the areas in which insights, garnered from good data, can best help the organization. This method will not only generate relevant questions but will facilitate a smooth, clear process.
Aggregate Data
After establishing parameters and questions that need answers, it's time to begin data collection. Here, decision-makers will determine which data sources will provide the most valuable insights to the organization.
Track Progress
This tried-and-true method for tracking the progress of the organization's data analysis is not a new one. Here, setting key performance indicators (KPIs) for both qualitative and quantitative data is in order. KPIs evaluate the performance of business activities, measuring the success of a company at reaching its operational and strategic goals, looking at different performance parameters.
Cull Irrelevant Data
In this method, the organization will use the KPIs above to exclude data that is considered to be irrelevant or extraneous. This will lead to a lean source of data that has the potential to be far richer in insights than the source data. Further, it facilitates a better focus of analytical efforts, leading to clearer insights.
Execute Statistical Analysis
One of the most comprehensive aspects of data analysis, this quantitative method enables the organization to drill down to the hard facts that will drive insights. Statistical analysis will center on such things as a cluster, regression, factor, cohort, data modeling, and predictive analytics.
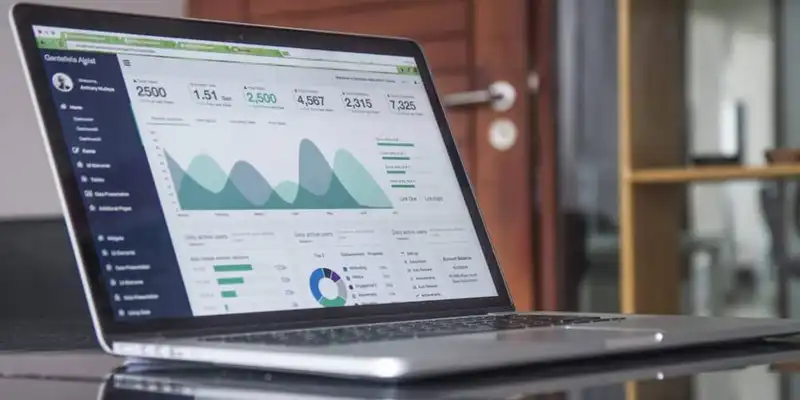
Employ Data Mining
Data mining is a method of data analysis that reveals patterns in large data sets using statistics, artificial intelligence (AI), and machine learning (ML). In data mining, large amounts of data can be processed in order to identify outliers and exclude these from the decision-making process.
Establish Data Management Practices
This method involves formulating systematized practices that help to efficiently manage, store, and utilize data within the organization. This will be specific to the organization, its operational goals, and the data sources in use. Once completed, the procedures should be scalable, and will help to make the overall analysis techniques function optimally.
Engage Technology
Integrating the right technology for core data analytics and statistical method data analysis is essential to the data analysis process. It's also an aspect that will probably be addressed fairly early in the process since in many cases, data sources will already have been identified.
While some organizations will be able to get by using Microsoft Excel or similar tools, others will require robust analysis platforms or business intelligence suites that facilitate the examination of critical data from valuable sources while working with dynamic inputs, such as KPIs.
Employ Data Visualization
Data visualization is powerful for two reasons- One, it is an all-encompassing method of analyzing data and two, it allows those who learn visually to derive insights from the data more effectively. An example of a data visualization tool would be an online dashboard that analyzes and displays various relevant metrics.
Constructing Narratives
Similar to data visualization, in the process of analyzing data and constructing insights, building narratives around what the data is telling the organization is a simple and straightforward way of imparting a vision to those conducting the analysis.
Once again, many people are visual learners, and storytelling (i.e., building a narrative) has proven to be an extremely efficient manner in which to impart information for millennia. In so doing, analytics can go from being largely incomprehensible to easily digestible.
Utilize Diagnostic Analysis
Diagnostic data analytics allow analysts and decision-makers to form an understanding of why events took place as they did during the course of business operations. There are two principal typesPredictive analytics, which uses data based on existing trends and past performance to predict likely outcomes, and Prescriptive analytics, which seek to develop responsive strategies based on a snapshot of the current state of an organization or department.
Consider Text Analysis
Business organizations generate quintillions of bytes of digital data every day. Much of this data is text-based, but unfortunately, much of this would (and definitely should) be relegated to the irrelevant data pile (see above). Tools for text analysis can aid in determining the relevance of text data from various sources, thus which data should be passed along for further analysis.
Among the additional resources that aid in data analysis includes Artificial Intelligence (AI) and Machine Learning (ML). These autonomous technologies are of course in ongoing development, and experts predict that these will play an increasing role in business and the development of emerging technologies in the coming years. These resources also make heavy use of text analysis.
Maintain Perspective
The utility of data analysis can be a double-edged sword, in that the sheer volumes of data have necessitated the continuous development of new methodologies, and will continue to do so as technology evolves and business needs change. This has given rise to even more being offered in the way of digital tools and suites designed for the express purpose of data discovery, intelligence and improvement.
Data analysis, data-driven paradigms and the myriad tools available to facilitate these processes have given rise to radical changes in organizations, and keeping up-to-date on processes and technical tools has become a full-time job for many managers and executives.
Some of these tools help organizations determine precisely what is relevant, which is no small task in itself. Out of all the data organizations aggregate, only around half of one percent is being analyzedbut with quintillions of bytes in the queue, it still represents an enormous amount of data.
Moving Forward
In short, data analysis encompasses the evaluation of data with statistical and other tools to discover information that can be beneficial to an organization. Knowing how to properly handle the data analysis process allows an organization to get the most from the data and make the right decisions.
As decision-makers examine insights derived from data, it is important that they consider both the statistical significance as well as the practical significance of the data. Of course, all of these are open to a certain degree of interpretation. This only reflects the fact that the human component remains inescapable, but in employing data analysis, an organization can count on creating continuously superior outcomes.