6 Different Types of Data Analysis to Look Out For
6 Types of Data Analysis
Data analysis refers to the process of examining, purifying, modifying, and modeling data to identify important information and solutions that help optimize problem-solving. Data analysis is included within data science, which refers to the analysis of information for various business reasons.
Data analysis is used in the business world to gain insight into inefficiencies, improve decision-making, and eliminate risks. By effectively performing data analysis, businesses can identify areas where waste occurs and use that information to achieve profitability. Here are the 6 types of data analytics, their purposes, and analysis techniques
1. Descriptive Analysis
The purpose of descriptive analysis is to describe a data set. This includes summarizing any primary analyses, measurements, and patterns.
If an organization is analyzing the sales of coats among females, a descriptive analysis may include a summary of the total cost sales among females, the months where sales were the highest, which areas of the country the sales were coming from, and whether there were any patterns discerned (i.e. more sales in January and February).
In short, descriptive analysis is a presentation and summary of all of the analysis data collected so far.
2. Exploratory Analysis
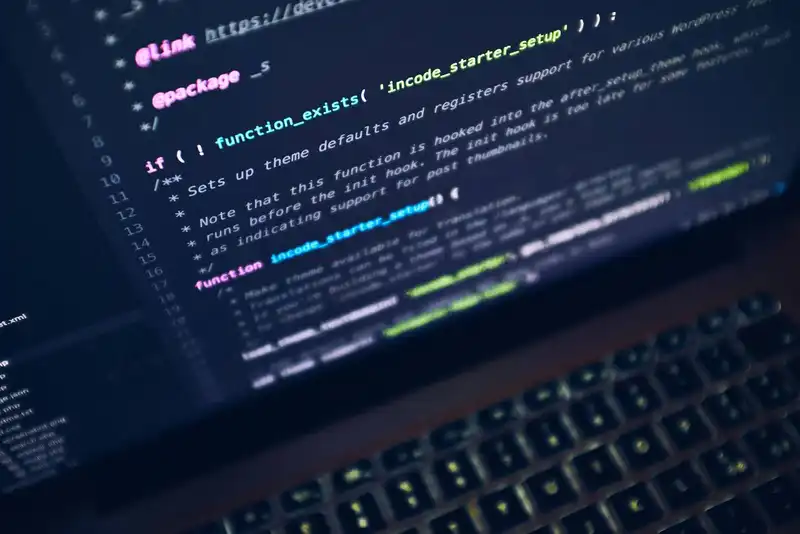
Exploratory analysis drills down into collected information to pinpoint correlations between variables that were formerly unseen. While relationships between variables do not imply causation, exploratory analysis can help to form a hypothesis about this relationship.
An exploratory analysis may assess why there were more chocolate sales in January and February vs. other months. Possible hypotheses include Valentine's day, relinquishing New Year's resolutions, or the cold weather.
3. Inferential Analysis
Inferential analysis takes a small portion of a data set to make inferences about a larger quantity of data. It uses estimated data and provides a standard deviation to make assumptions about a larger group. While these inferences are never entirely accurate, they are more reliable if the sample size is representative of the larger population.
For example, an organization may look at 30 out of 500 customer surveys and find that poor customer service is a common complaint. Because of this regular theme throughout the 30 surveys collected, the company assumes that customer service problems are a concern for the remaining 470 feedback reports, and probably for the entirety of their customers.
In summary, IA helps an organization make inferences and predictions about future patterns by displaying patterns in small samples.
4. Predictive Analysis
Predictive analysis uses historical and real-time information to find trends that help to forecast future outcomes. The reliability of these predictions is based on the type of data collected and the models used.
It's important to note that one variable predicting another variable doesn't necessarily imply that there is a cause and effect relationship between the two. There needs to be a larger, more in-depth analysis of variables to pinpoint whether there is a correlation.
To illustrate, a business sees in its data that sales have been low for the past 3 years during June, July, and March. An analyst can predict that sales will probably be low for the next year during these months unless they run a promotion, change the type of products sold, or perform another action that changes the end result.
5. Causal Analysis
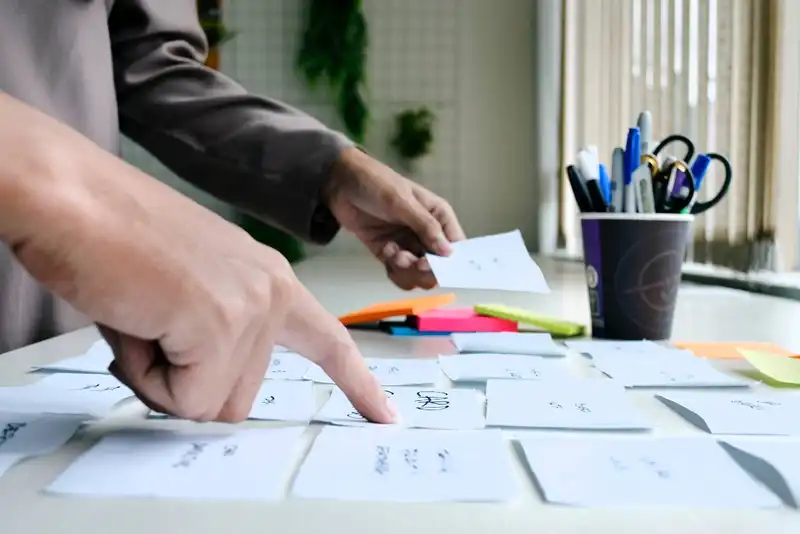
Causal analysis takes a set of variables and assesses whether there is a cause and effect relationship between them and why. To identify the cause, an analyst should determine whether the hypothesized relationship leads to the desired outcome.
Simply viewing the data without performing in-depth analysis will not help find the underlying reason behind the relationship.
For example, a drug manufacturer wants to test how well a new drug improves attention in young adults. It compares a data set of young adults in the United States vs. the individuals receiving the drug in a trial study and then assesses how the new drug impacts attention.
6. Mechanistic Analysis
The purpose of the mechanistic analysis is to comprehend the precise fluctuations in data that result in fluctuations in other data.
This type of analysis is usually applied in circumstances that necessitate accuracy and very little room for mistakes. The medical industry, engineers, and the scientific community primarily use mechanistic analysis to test the safety and effectiveness of a product.
To illustrate, a medical device company wants to test how effective a dialysis machine is. A mechanistic analysis encompasses the exact control of data (both controlling and manipulative variables) by accurately measuring each data set and the desired results.
The overarching strategy used to perform a mechanistic analysis is how the medical and scientific community provides developments that lead to progress in society.
Key Takeaways
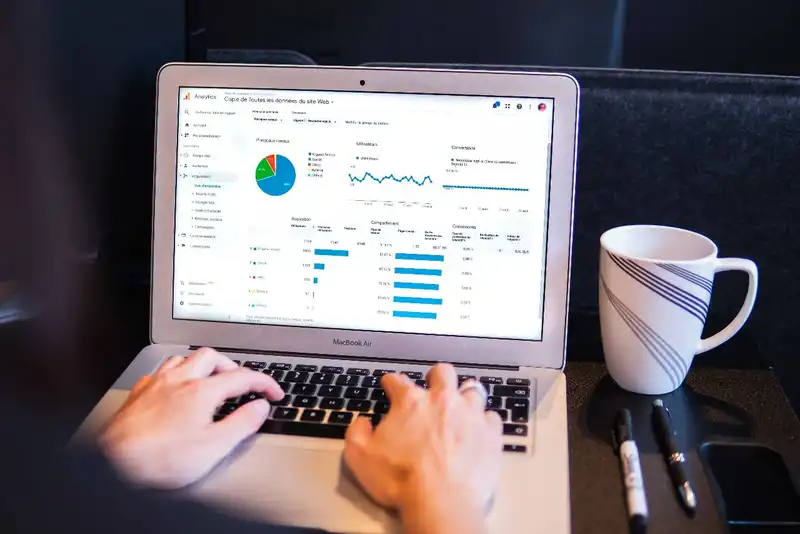
In conclusion, here are the 6 types of data analysis used by various industries
- The descriptive analysis summarizes a set of data, including measurements and patterns. Exploratory analysis tools drill down into collected data to identify any relationships between data sets.
- The inferential analysis takes a small portion of data and uses it to assume about a larger population.
- Predictive analysis uses historical and real-time data to forecast future trends. The causal analysis takes two variables and determines whether there is a relationship between them and why.
- The mechanistic analysis method is used by the scientific and medical community to see whether a fluctuation in data causes fluctuations in other data.