Gaining Insight into Data Integration
What is Data Integration?
Data integration utilizes technical and business processes for combining data from different sources to supply an integrated data view. The consolidation of disparate sources of data into a single dataset is incredibly beneficial for business processes. In fact, data integration can assist with virtually any business process data is involved in. Unsurprisingly, data integration is considered an essential data management process and a common data related business priority. The big data integration process is commonly compared to the data integration process due to its increasing popularity. Also similar to the big data integration process, the data integration process combats common challenges data and analytics leaders face.
Data integration allows users to access data across source systems consistently. Consistent data access and delivery of data across source systems benefits business processes significantly. Data integration has been even further optimized through the development of data integration platform and software programs. An integration platform facilitates automated data integration seamlessly. An integration platform also route and connect data from source systems to target systems via powerful data integration techniques.
Common data integration techniques make it possible for businesses to access data insights full of valuable information. Techniques range from the extract, transform, and load to data replication process. Data replication and data virtualization are common data integration techniques. Data replication copies data from one database to another. Data replication keeps data syncronized for operation usages and as a backup. Data virtualization consists of combining data from different systems for a unified view. Data virtualization avoids the need to load data into a new repository.
An important data integration technique distinction is extract, transform and load compared to extract, load, and transform. In extract, transform and load copies of disparate sources of data are collected into a data warehouse or database. In extract, load, and transform data loads into a big data system and is not translated unless data analytics necessitates.
Streaming data integration and change data capture are other common data integration techniques. Streaming data integration is a real time method for integrating different data streams collectively. Real time data integration feeds the different data streams into data repositories and analytics systems. Change data capture is used to identify real time data changes. With change data capture, real time changes are applied to the data warehouse or any other repository utilized.
The Importance of Data Integration
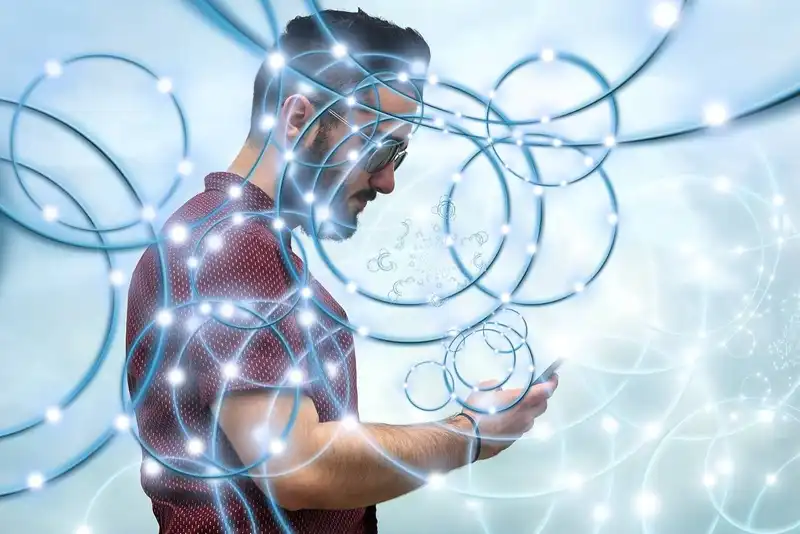
As enterprise data becomes increasingly complex, data integration is more important than ever before. A data intergration project reveals both historical and near real time enterprise data for analysis. Data integration extracts valuable data insights utilized for everything from real time decision making to future business processes developed. Data integration assures that the time data related processes require is spent most efficiently. For example, business professionals can use internal data for presentations instead of needing to use case studies from external sources.
Not only is data integration useful for understanding current business operations but it also helps predict future events. For example, a data intergration project could reveal upcoming marketplace threats or opportunities. With this knowledge, businesses can prepare for upcoming events most effectively. As such, data intergration is an excellent risk management tool with very real bottom line implications. Beyond internal enterprise application, data integration is often essential for external collaborations. Collaborating with various suppliers or business partners is much easier with excellent data intergration.
A data integration solution also combats pesky legacy system issues. A legacy system is defined as any outdated hardware or computing software. The ability to access data on legacy systems supports data management best practices. A proper data integration solution allows legacy data use in contemporary business intelligence applications. As such, data integration measurably saves time and improves data access for businesses.
How Data Integration Benefits Business
Data integration significantly benefits both business processes and business operations overall. As such, many businesses consider a data integration project well worth the resources required. Various notable ways data integration benefits businesses include-
1. Time Saving
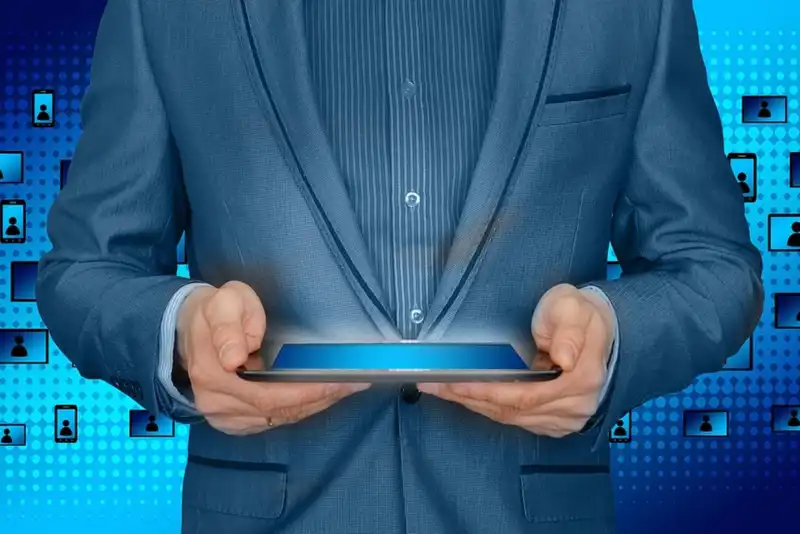
The time required to manually process data is excessive and inefficient. Time savings related to data integration span across business processes and departments. For example, the right data integration tools can decrease data science hand coding requirements. Alternatively, a unified view of data allows decision making business professionals to reference real time and historical data quickly. As a result, data integration saves valuable time that can be utilized instead towards more productive goals.
2. Data Quality
Data quality is generally classified as a top data management consideration. Data integration ensures data quality by identifying any data quality issues. Integration tools can even perform essential data quality improvements. As a result, not only is data quality optimized but data analytics processes as well.
3. Collaboration
Proper collaboration is an essential component of any successful business. Data integration supplies a unified view of both real time and historical data for maximum collaborative potential. Cloud data availability is especially important for remote workers that need to collaborate with onsite workers. A typical analytical use case is notably benefited by unified data availability. Without unified data available an analytics use case requires extensive efforts to process data correctly. An analytics use case without unified data may entail laborious data reformatting and cleansing.
5 Necessary Data Integration Strategies
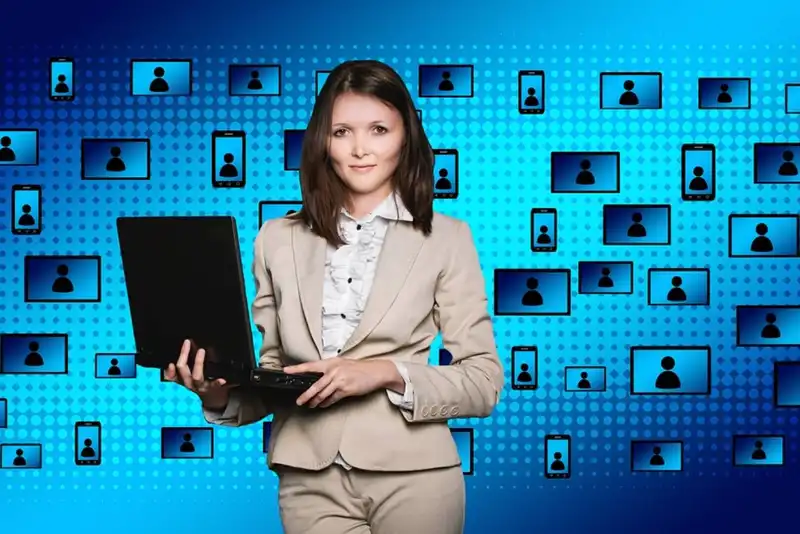
There are various different data integration strategies available for business utilization. Decision making initiatives regarding various data integration strategies are benefited by a comprehensive understanding of different data integration strategies available. Decision making should also consider factors such as business size and resources available for data integration. Although decision making may require extensive effort, data integration business decisions are well worth the effort. 5 different data integration strategies that all businesses should know include-
1. Common Storage
Common storage integration is the most frequently utilized storage application within data integration. Common storage integration is also the underlying principle behind a traditional data warehouse solution. In common storage integration, a data replication of original source data is held in the integrated system. As a result, common storage integration supplies a unified view of processed, copied data.
2. Uniform Access
Uniform access integration is a data integration type focal on producing a front end that appears consistent. The consistent front end appearance must be accessible from different sources for uniform access integration success. It is important to note that the data is left within its original source in uniform access integration. The appearance of front end consistency between different source systems is more easily accomplished through object oriented database management systems.
3. Application Based
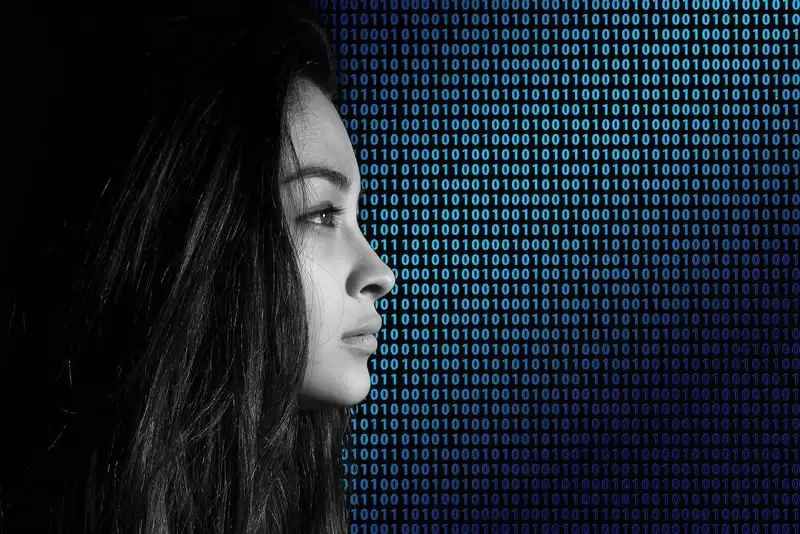
Application based integration is a data integration type that focuses on compatibility. Software applications find, collect, and integrate data during application based integration. The data integration stage of application based integration necessitates combining different sources systems and disparate sources of data. A successful data integration project necessitates making data from different sources compatible with one another. Source system compatibility enables data transmission from one source system to another.
4. Middleware Data
Middleware data integration is a data integration type that focuses on mediation. Acting as a mediator, the middleware application assists to normalize data. The objective of normalizing data is to transport it into the master data pool. Middleware is a good option for when data access from an application is unavailable.
5. Manual Data
Manual data integration is widely considered an inefficient data integration type. Manual data integration, as its name suggests, is the process by which a user collects data manually. The user must access, clean, and combine data into a data warehouse throughout this process. As such, manual data integration is not an integration solution that is generally recommended.
5 Challenges with Data Integration
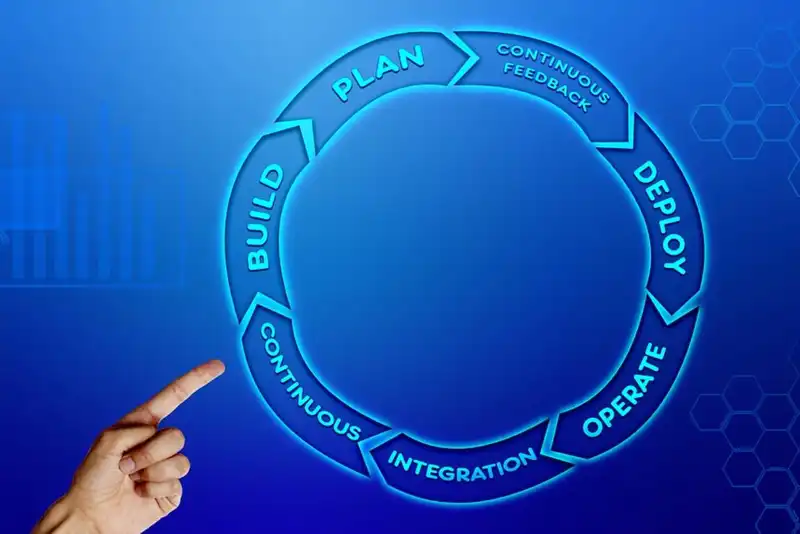
Data integration is a very technical business challenge that brings its own set of concerns. Thankfully, recognizing challenges data integration commonly carries is a great way to prepare for or even avoid them. Common challenges data integration system building carries for businesses include-
1. Legacy
Legacy systems are often a significant integration process challenge. Although legacy systems still serve their original function they do not support growth. As such the new system and legacy systems data are incompatible.
An example of a common legacy systems data issue is a time data marker. The date and time data collection occurred may not be accurate or even annotated in a legacy system. If the new system necessitated a time data annotation and the legacy system could not supply it, challenges arise. As such, any new system added in the future should include real time data capabilities to avoid later issues.
2. Route
A successful integration solution is unlikely if not impossible without a great plan. Too often, an integration solution is pursued before a solid integration process plan is established. To seek an integration solution without integration process steps can create many challenges. Similar to other business processes it is essential that data integration is well planned and organized. From previous data migration efforts to new integration tool investments, there are various integration project particulars to consider.
3. External
External data poses its own challenges to data integration processes. If external data has more detail or less detail than internal data sources issues are likely to arise. It can be difficult to access data and combine data that contain different levels of detail. As such, any data source from external vendors can create data analytics and integration challenges.
4. Maintenance
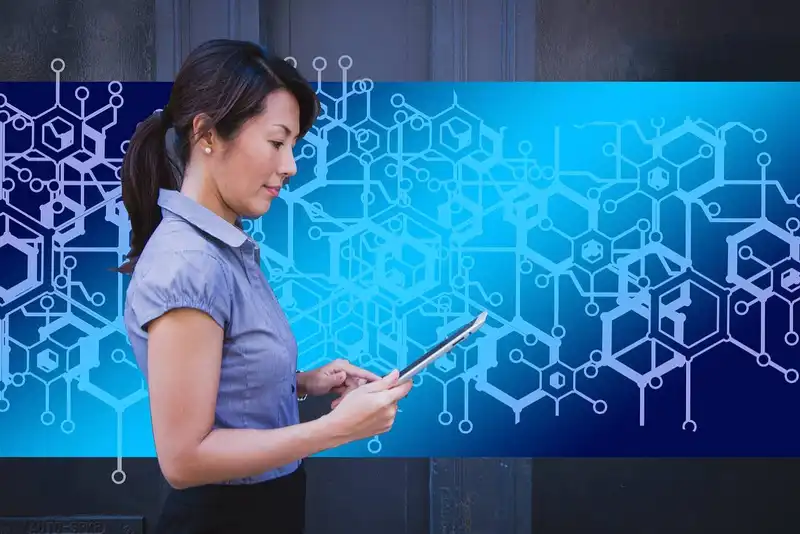
Too often businesses look to integrate data without considering ongoing maintenance. Data integration efforts must keep up with best practices and data management advancements. Any proper integration process is ongoing, welcoming future data governance and data quality advancements.
5. Change
A new system will generate different data types which can bring challenges. Different data types may include real time or unstructured data from various sources. Different sources range from cloud data to enterprise data collected from sensors. Different data volume, speed, and new system format often challenges data integration. Thankfully there are solutions for common challenges data integration brings.
Data Integration Features to Look Out For
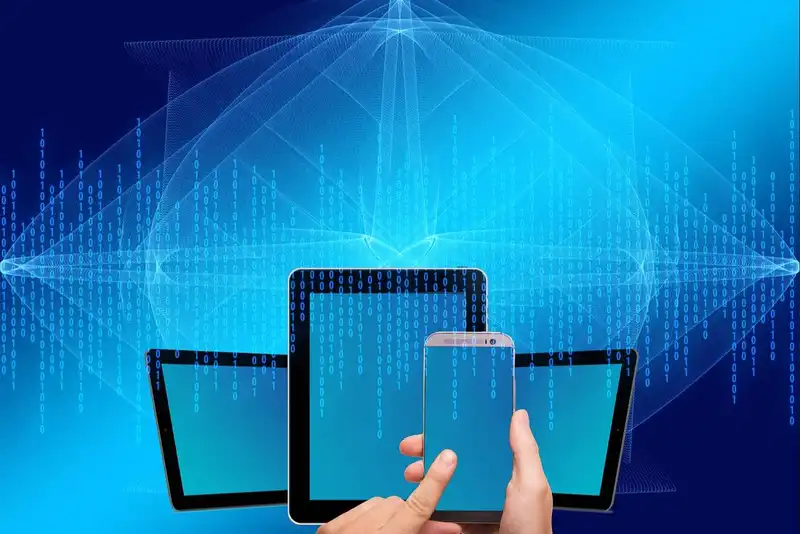
Integration tools combat many common challenges data integration processes contain. Integration tools also simplify and streamline the integration process noticeably. It is helpful to understand which features to look for in a data integration tool or integration platform solution. From open source architectures to cloud compatibility, there is much for businesses to consider!
Ease of usage is a top consideration for integration process tools. An integration platform or integration solution should include easy to learn data integration solutions like a graphic user interface. A graphic user interface makes data visualization throughout the data pipeline much simpler. Cloud compatibility simplifies everything from efforts to view data to combine data easier. Cloud data compatibility in a integration tool may be a single, multi, or hybrid cloud environment. Especially with hybrid cloud data models, portability is also crucial to make data available from any location.
Open source architectures generally supply additional flexibility and avoid vendor lock ins. Open source advocates may use cases where data storage or data management vendors didn't deliver the customer experience desired. While some open source advocates will use case by case examples, open source may not always be the best option. Any paid integration tool invested in should have a transparent price model. Businesses may use case study research or customer experience testimonials to learn more about specific integration tool vendors.
Conclusion for Data Integration
- Proper data integration is absolutely essential for business intelligence and insights.
- Necessary data integration strategies include common storage, uniform access, application base, middleware data, and manual data.
- Challenges common in data integration range from legacy systems to external data sources.
- Ease of usage and open source architectures are both data integration features to look out for.