Data Integrity vs Data Quality- Definitions & Differences
Data Integrity vs Data Quality
Both data integrity and data quality should absolutely be considered top business priorities. Business data is a strategic asset that is invaluable for a wide range of initiatives, from decision making to forecasting.
As such, both ensuring data quality and maintaining data integrity is imperative. Without maintaining data integrity or ensuring data quality businesses expose themselves to a variety of risks. Bad data results in increased human error in decision making and other data driven business processes. Discussing data integrity vs data quality helps businesses avoid bad data and human error while advancing data management best practices.
Unfortunately, the term data integrity and the term data quality are incorrectly used interchangeably very often. The conflation between data quality and data integrity is understandable as both terms describe the condition of data. However, data integrity and data quality are two separate terms with their own unique definitions. As such, both the similarity and difference data quality and data integrity have are important for businesses to consider.
Data Quality
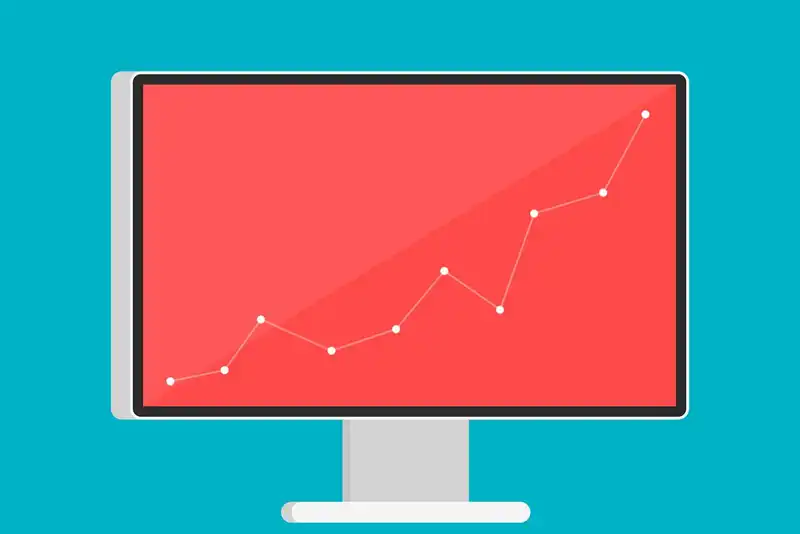
In regards to data quality, there are actually various definitions of the term, depending on whom you ask. Broadly, data quality refers to the state of quantitative and qualitative information. Data quality is also commonly defined as the reliability of data.
There is also a notable difference between data quality and quality data definitions. For data to be regarded as quality it must also adhere to strict criteria. The criteria for quality data include completeness, uniqueness, validity, timeliness, and consistency. Without meeting all the criteria, quality data is not assured.
Even conversations about what constitutes high quality data can be complicated. For data to be considered high quality it must fit its intended purpose of use. For example, to ensure data used for decision making initiatives is high quality, it must fit. High quality data also must accurately exemplify the real world construct it describes.
There are various situations in which the different definitions of high quality may contradict each other. For example, a customer master data record may be adequate for issuing an invoice upon receipt of payment. However, the same customer data record may not be complete or correct for customer service. The proportionality and profitability of ensuring real world alignment of high quality data is an important business decision making consideration. As such, a balance between the definitions of high quality data is often pursued in real world practice.
Data Integrity
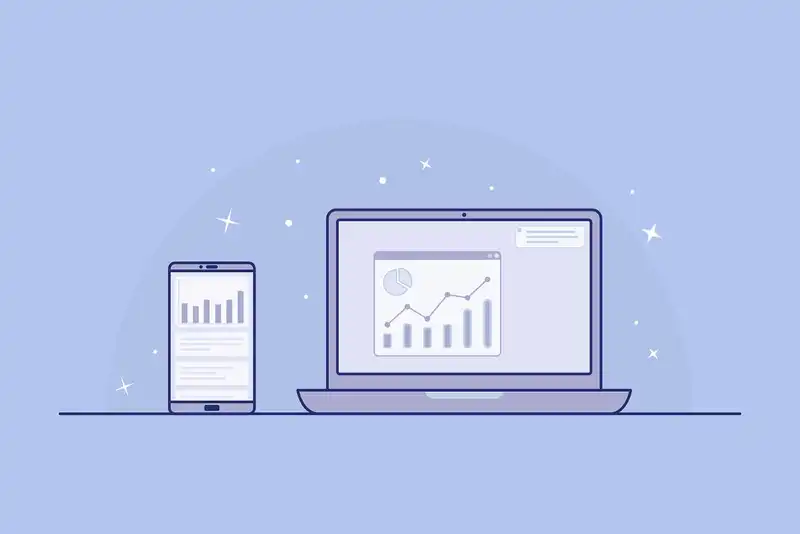
If average people were asked about how they defined integrity the answers would likely be similar. However, defining data integrity would presumably be more challenging and result in many different responses. Data integrity refers to the validity, completeness, consistency, and accuracy of data. Data integrity encompasses these qualities throughout data lifecycles. Additionally, data integrity refers to data security and safety regarding regulatory compliance.
There are 4 primary pillars of data integrity that all business professionals should be aware of. Data integration is a data integrity important pillar necessary for optimal visibility. Data integration collects business data from various sources in order to supply users with a unified view.
Data integration sources can range from a cloud data warehouse to legacy systems. Data quality is another data integrity important pillar. In order to be considered helpful for decision-making initiatives, data quality must be valid, unique, complete, timely, and consistent.
Location intelligence and data enrichment are also data integrity important pillars. Data enrichment enhances internal data with added meaning, context, and nuance by utilizing data from external sources. Adding additional information from external sources produces business data that is more comprehensive and contextualized.
By using business data that is more contextualized and comprehensive, companies can also maintain data analysis that is more powerful. Location intelligence uses location insight and data analytics to make data more actionable. As a result of location intelligence data is not only more actionable but also richer.
Differences Between Data Integrity vs Data Quality
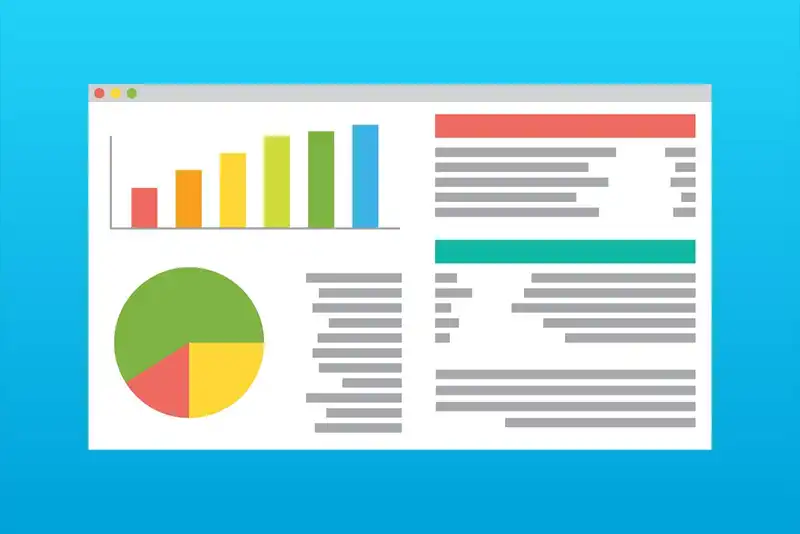
The framing of data integrity vs data quality is actually quite misleading in itself. In actuality, data quality is an aspect of data integrity. As data integrity contains data quality as a component, the two are intertwined. Simply put, data quality is a subcomponent of data integrity.
Another significant difference data integrity and data quality have is the breadth of their reach. Data quality is generally considered an excellent place to begin. Alternatively, data integrity enhances the usefulness data offers a business.
As such, instead of contemplating data quality vs data integrity, businesses should contemplate using both data integrity and data quality. The combination of data integrity and data quality enhances everything from decision making to data management overall. Ultimately, businesses proactively ensuring data quality and defined integrity observe benefits in all data driven initiatives.
Key Takeaways for Data Integrity vs Data Quality
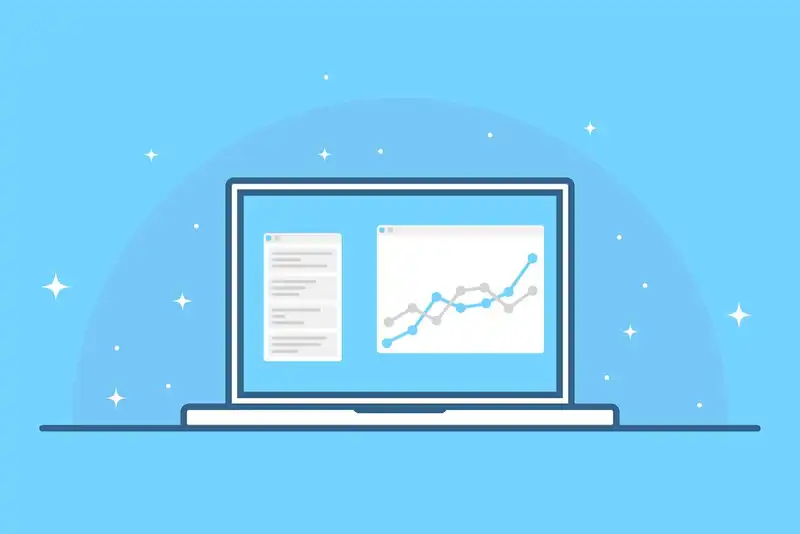
- Business professionals must differentiate between data quality and data integrity.
- Data quality is a component of data integrity and as such the two are closely intertwined.